Introduction
The Role Of Artificial Intelligence In Modern Hacking Techniques
The Role of Artificial Intelligence in Modern Hacking Techniques explores the intersection of AI and cyber threats, including AI-driven attacks like automated exploitation and intelligent phishing. The article delves into defensive AI measures and ethical hacking, highlighting the need for proactive cybersecurity strategies to counter evolving threats. It also anticipates future trends, emphasizing the importance of global collaboration and responsible AI practices in safeguarding digital ecosystems.
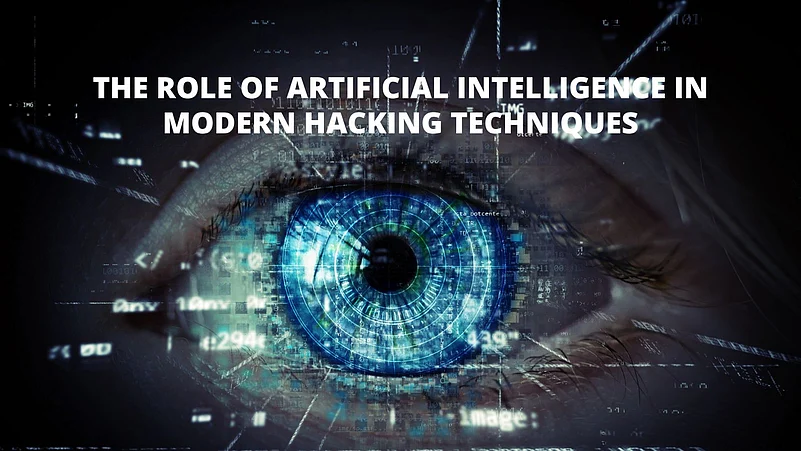
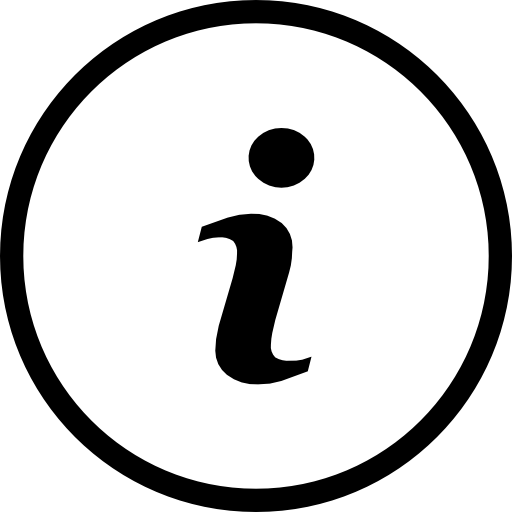
Definition of Artificial Intelligence (AI): Artificial Intelligence, commonly known as AI, encompasses the field of computer science dedicated to creating systems capable of carrying out tasks that traditionally rely on human intelligence. These tasks span a wide spectrum, ranging from problem-solving, speech recognition, learning, to decision-making processes. AI systems possess the ability to analyze data, recognize patterns, and adjust their behavior based on accumulated experience. This versatility makes AI a potent and multifaceted tool applicable across diverse domains, contributing to advancements in technology, research, and various industries. The fundamental goal of AI is to emulate and, in some cases, exceed human cognitive functions, opening up new possibilities for innovation and automation in an ever-evolving technological landscape.
Evolution of Hacking Techniques: The evolution of hacking techniques parallels the advancements in technology and connectivity. Initially, hacking primarily involved simple exploits and unauthorized access to systems. With the growth of the internet, hacking evolved into more sophisticated methods, including malware, phishing, and social engineering. As security measures improved, hackers adapted by employing more intricate tactics, such as exploiting software vulnerabilities and leveraging interconnected systems.
Intersection of AI and Hacking: The intersection of AI and hacking represents a paradigm shift in cyber threats. Hackers now leverage AI to enhance the efficiency and effectiveness of their attacks. AI enables automation of various tasks, making attacks faster and more scalable. Machine learning algorithms are employed to analyze vast amounts of data, allowing hackers to identify vulnerabilities, craft personalized phishing messages, and adapt their strategies based on the target's defenses. The symbiotic relationship between AI and hacking introduces new challenges for cybersecurity, as defenders must harness AI for protection while staying ahead of AI-driven threats. This dynamic interplay has reshaped the landscape of cyber warfare, marking a crucial era in the ongoing cat-and-mouse game between security professionals and malicious actors.
Overview of AI-driven Attacks
Artificial Intelligence (AI) has significantly transformed the landscape of cyber attacks, ushering in a new era where malicious actors leverage advanced algorithms and machine learning to conduct more sophisticated and efficient campaigns. This section will provide an in-depth look at the key elements characterizing AI-driven attacks.
Automated Exploitation:
AI-driven automated exploitation represents a paradigm shift in the speed and scale of cyber attacks. Automated tools powered by AI can identify vulnerabilities in software systems, networks, or even IoT devices at a pace unmatched by traditional methods. These tools continuously scan for weaknesses, assess potential targets, and exploit vulnerabilities rapidly. The automation of the exploitation process allows attackers to launch coordinated and widespread attacks, making it challenging for defenders to keep up.
Intelligent Phishing:
Intelligent phishing attacks leverage AI to craft highly targeted and convincing messages, significantly increasing their chances of success. Traditional phishing relied on generic messages sent en masse, hoping to lure unsuspecting individuals. AI algorithms now enable attackers to analyze vast datasets, including social media profiles and online behaviors, to create personalized and contextually relevant phishing emails. This level of sophistication makes it difficult for individuals to discern between genuine and malicious communications, amplifying the effectiveness of phishing campaigns. Moreover, AI can facilitate real-time adaptation of phishing strategies based on the recipient's responses, making these attacks dynamic and harder to detect.
These AI-driven attack techniques pose serious challenges to traditional cybersecurity measures. As attackers increasingly incorporate automation and intelligence into their methods, defenders must adopt more advanced and adaptive security solutions to thwart these evolving threats. The arms race between AI-driven cyber attacks and defense mechanisms underscores the need for a proactive and resilient cybersecurity strategy in the face of this rapidly changing threat landscape.
Case Studies: Notable Examples of AI in Cyber Attacks
Deepfake Social Engineering:
Deepfake technology, powered by sophisticated AI algorithms, has been weaponized for social engineering attacks, marking a significant threat in the realm of cyber deception.
Case Study:
One notable instance involves a deepfake-powered social engineering attack on a high-profile individual. In this case, an attacker used AI-generated audio and video to replicate the target's voice, facial expressions, and mannerisms convincingly. The assailant then employed these deepfake elements in a carefully crafted phishing campaign. The target, believing the communication was legitimate due to the highly realistic nature of the deepfake, inadvertently disclosed sensitive information.
Implications:
Deepfake social engineering attacks pose serious risks, as they can manipulate trust and exploit human vulnerabilities. The ability to create indistinguishable replicas of individuals raises concerns about the authenticity of digital communication and highlights the need for enhanced verification measures in online interactions.
Adversarial Machine Learning:
Adversarial Machine Learning involves manipulating AI systems by introducing carefully crafted input data to deceive the model's decision-making process. This technique is employed to subvert the integrity of AI-powered defenses.
Case Study:
In a real-world scenario, an organization utilized an AI-driven intrusion detection system to identify malicious network activity. However, attackers implemented adversarial machine learning techniques by subtly modifying the patterns of their malicious actions to evade detection. By exploiting vulnerabilities in the AI model's decision boundaries, the attackers successfully deceived the intrusion detection system, allowing them to carry out their activities undetected.
Implications:
Adversarial Machine Learning underscores the vulnerability of AI systems to manipulation. As AI becomes integral to cybersecurity defenses, understanding and mitigating adversarial attacks are crucial. This case study emphasizes the need for continuous improvement in AI model robustness and the development of countermeasures to defend against evolving adversarial tactics.
These case studies highlight the tangible and evolving threats posed by AI in cyber attacks. As technology advances, the cybersecurity community must remain vigilant, adapting defenses to stay ahead of sophisticated AI-driven tactics employed by malicious actors.
Defensive AI Measures
AI in Cybersecurity
Intrusion Detection Systems (IDS):
Intrusion Detection Systems (IDS) are a fundamental component of cybersecurity that utilizes AI to monitor network or system activities for signs of malicious behavior or unauthorized access.
Implementation:
AI-powered IDS leverage machine learning algorithms to analyze patterns in network traffic and user behavior. By learning what is considered normal, these systems can detect anomalies indicative of a potential security threat. This proactive approach allows for the rapid identification of suspicious activities, enabling security teams to respond swiftly to mitigate potential breaches.
Behavioral Analysis:
Behavioral analysis involves the use of AI to assess and understand the normal patterns of behavior within a network or system. Deviations from established norms can signal potential security incidents.
Implementation:
AI algorithms continuously analyze user behavior, application interactions, and network traffic. By learning patterns over time, the system can identify unusual activities that may indicate a security threat. Behavioral analysis adds a layer of sophistication to cybersecurity defenses, enabling the detection of insider threats, zero-day attacks, and other anomalies that may go unnoticed by traditional security measures.
Challenges in Defending Against AI-Enhanced Attacks
Speed and Automation:
Challenge:
AI-enhanced attacks operate at unprecedented speeds, exploiting vulnerabilities and adapting to changing conditions in real-time. The automated nature of these attacks allows malicious actors to launch large-scale, coordinated offensives with minimal human intervention.
Defensive Response:
Defending against AI-driven attacks requires cybersecurity professionals to deploy AI and machine learning in their defensive strategies. Automated threat detection and response mechanisms must match the speed of AI attacks to effectively neutralize threats in real-time. This necessitates the integration of AI technologies into security operations to create a dynamic and responsive defense posture.
Adapting to Evolving Tactics:
Challenge:
AI-driven attacks continuously evolve as attackers leverage adaptive algorithms to bypass traditional security measures. The ability of AI to learn and adjust strategies in real-time poses a constant challenge for cybersecurity defenders.
Defensive Response:
Cybersecurity professionals must adopt a proactive stance by continually updating and enhancing their AI models. Regularly training AI systems with new data to recognize emerging threats, combined with threat intelligence sharing within the cybersecurity community, helps defenders stay ahead of evolving tactics. Additionally, fostering a culture of collaboration and knowledge exchange is crucial for developing effective countermeasures against the rapidly changing landscape of AI-enhanced attacks.
In navigating the complex landscape of cybersecurity, leveraging AI as both a defensive and offensive tool is essential. As technology evolves, the integration of advanced AI measures becomes paramount to maintaining robust and adaptive cybersecurity defenses.
Ethical Hacking with AI
Ethical hacking, also known as penetration testing or white-hat hacking, involves authorized and controlled attempts to exploit system vulnerabilities for the purpose of identifying and fixing security weaknesses. Ethical hackers use their skills and knowledge to simulate real-world cyber attacks, providing valuable insights to organizations on potential risks and areas for improvement in their security posture.
Objectives:
The primary objectives of ethical hacking include assessing the security of information systems, identifying vulnerabilities, and recommending appropriate countermeasures. Ethical hackers act as cybersecurity professionals who think like malicious attackers, employing various tools and techniques to uncover potential weaknesses in a system.
AI Tools for Security Professionals:
1. Vulnerability Scanning:
Vulnerability scanning is a crucial aspect of ethical hack that involves the use of AI-powered tools to identify and assess potential weaknesses within a system, network, or application.
AI Implementation:
AI-enhanced vulnerability scanning tools utilize machine learning algorithms to recognize patterns associated with known vulnerabilities. These tools automate the scanning process, efficiently identifying and prioritizing potential weaknesses based on the level of risk they pose. AI also enables these tools to adapt and evolve, learning from new vulnerabilities as they emerge.
Benefits:
Efficiency: AI accelerates the scanning process, allowing for rapid identification of vulnerabilities.
Accuracy: Machine learning algorithms enhance the accuracy of identifying vulnerabilities, reducing false positives and negatives.
Adaptability: AI-powered tools can adapt to changing threat landscapes, ensuring continuous effectiveness over time.
2. Threat Intelligence:
Threat intelligence involves gathering and analyzing information about potential cyber threats to understand the tactics, techniques, and procedures employed by malicious actors.
AI Implementation:
AI is instrumental in processing vast amounts of data quickly, making it ideal for analyzing threat intelligence. AI tools can sift through large datasets to identify patterns, correlate information, and predict potential threats. Machine learning algorithms can learn from historical data, enabling the identification of emerging threats based on evolving attack patterns.
Benefits:
Proactive Defense: AI-driven threat intelligence enables security professionals to anticipate and proactively defend against emerging threats like Pirater.
Real-time Analysis: AI tools can provide real-time analysis of threat data, allowing for immediate response to potential security incidents.
Scalability: AI enhances the scalability of threat intelligence operations, enabling organizations to handle large volumes of data efficiently.
Ethical hacking, empowered by AI tools, plays a crucial role in fortifying cybersecurity defenses by identifying vulnerabilities, understanding evolving threats, and ensuring organizations are well-prepared to face potential cyber attacks.
Future Trends
The Continuous Evolution of AI and Hacking:
The future of AI and hacking promises to be dynamic, marked by the perpetual evolution of both offensive and defensive capabilities. This section explores the anticipated trajectories of AI development and its implications for hacking techniques.
AI Advancements:
As AI technologies continue to advance, hackers are likely to incorporate more sophisticated algorithms and machine learning models into their attack strategies. This evolution may lead to the creation of AI-driven attacks that can adapt in real-time, making them highly elusive and challenging to counter. Conversely, defensive AI measures will also progress, introducing more intelligent and automated systems to detect, prevent, and respond to cyber threats.
Automation and Autonomous Agents:
The integration of automation and autonomous agents in hacking tools is expected to increase, allowing attackers to scale their operations with minimal manual intervention. Autonomous agents equipped with AI capabilities could autonomously identify vulnerabilities, craft tailored attacks, and execute them without direct human involvement, posing significant challenges for traditional security measures.
AI-Enhanced Social Engineering:
Social engineering attacks, powered by AI, may become more personalized and convincing. AI algorithms can analyze vast amounts of personal data to tailor phishing messages and manipulate human behavior effectively. This raises concerns about the exploitation of individuals' trust through highly convincing AI-generated content.
Potential Scenarios and Impacts:
Emergence of AI-Only Threats:
One potential scenario is the emergence of AI-only threats, where malicious entities deploy AI-driven attacks that are beyond the capabilities of conventional security measures. Such threats might exploit zero-day vulnerabilities, create highly realistic deepfake content for manipulation, or launch large-scale coordinated attacks with minimal human intervention.
Increased Sophistication of Defensive AI:
In response to evolving threats, defensive AI measures are likely to become more sophisticated. AI-driven security systems will leverage advanced analytics, machine learning, and automation to adapt to the rapidly changing threat landscape. This could involve the use of predictive analytics to anticipate potential attack vectors and proactively implement countermeasures.
Ethical Considerations:
The future trends in AI and hacking also raise ethical considerations. Striking a balance between harnessing AI for ethical hacking purposes and preventing malicious use is crucial. The development and implementation of ethical guidelines, regulations, and responsible AI practices will become increasingly important to ensure the responsible use of AI in cybersecurity.
Global Collaboration:
As AI-driven cyber threats become more complex and cross-border, global collaboration among cybersecurity professionals, organizations, and governments will be essential. Sharing threat intelligence, best practices, and jointly developing countermeasures will be crucial in staying ahead of emerging cyber threats.
In navigating the future landscape of AI and hacking, continuous vigilance, innovation, and collaboration will be imperative. The integration of AI into both offensive and defensive cybersecurity measures will shape the dynamics of cyber warfare, requiring adaptive strategies to effectively mitigate emerging risks.
Conclusion:
In conclusion, the interplay between Artificial Intelligence (AI) and modern hacking has ushered in a new era of dynamic and sophisticated cyber threats. The evolution of AI-driven attacks, such as automated exploitation and intelligent phishing, highlights the agility and adaptability that malicious actors now possess. As explored in this discussion, defensive measures, including AI in cybersecurity, vulnerability scanning, and behavioral analysis, play crucial roles in mitigating these evolving threats. The future trends indicate a continuous arms race, with AI advancements influencing both offensive and defensive capabilities. To fortify our digital defenses, there is a pressing need for a proactive stance in cybersecurity preparedness. This involves not only staying abreast of the latest AI-driven threats but also fostering global collaboration, implementing ethical hacking practices, and advancing the development of responsible AI frameworks. As we navigate this intricate landscape, a collective call to action for enhanced cybersecurity preparedness is paramount to safeguarding the integrity and security of our digital ecosystems. The ongoing synergy between AI and hacking demands a united and vigilant effort to ensure a resilient defense against emerging cyber threats.
Disclaimer: The above is a sponsored post, the views expressed are those of the sponsor/author and do not represent the stand and views of Outlook Editorial.
- Previous StoryGrooming The Fashionable: Mohit Khandelwal's Journey From Passion To Influence
- Next Story